基于过去3年内对提交给《柳叶刀》的1000多篇论文进行审核的总结,发现了这些在科研结论中常见的统计使用错误,并给出了规避错误的建议。
描述性统计对于理解数据至关重要,对于定量变量选择何种描述性统计指标描述,根据数据分布情况而定,可选择报告均值和标准差,或者报告中位数和四分位数范围(IQR)。同时,需要提供分析中使用的变量的直方图或表格等补充资料。
所有统计分析都建立在基本假设的基础上,如果可行的话,最好使用图形检查所有模型假设。
显示精确的p值,例如,0.032的p值应显示为p=0.032,而不是p<0.05,然而,可以使用不等式p<0.0001来报告非常小的p值。
不要简单将结果描述为没有效果,除非区间估计的所有效应值均没有临床意义。
基于临床重要性解释结果,并使用95%置信区间适当估计关联。
根据背景信息识别混杂因素,而不是统计学检验。
如果缺失数据的比例很高,可能会影响结果,不要简单删除不完整数据,建议使用逆概率加权或多重插补等方法。
使用专用的方法评估和处理稀疏数据偏倚(sparse-data bias)。
如果结果发生率较高,应报告危险比(risk ratio)或危险差(risk difference),而不是比值比(odds ratio)。
即使使用了相乘模型(multiplicative),也要评估相加交互作用(additive interactions)。
英文原文:
Panel: Basic recommendations for accurate reporting ofstatistics
Depending on the distribution, report either mean and SD or median and lQR for the description of quantitative variables. Provide supplemental material showing histograms or tables of the variables used in analyses.
Check all model assumptions, preferably with graphs where feasible.
Do not dichotomise p values ≥0.0001; instead, show the precise p value (eg, a p value of 0.032 should be shown as p=0.032, not p<0.05). However, the inequality p<0.0001 can be used to report very small p values.
Do not report results as showing no effect, unless all effects inside the interval estimate are clinically unimportant.
Interpret results on the basis of the clinical importance, with appropriate estimates of association with 95% Cls.
ldentify confounders on the basis of background information,as depicted in causal directed acycic graphs, not signifcance tests.
If the proportion of missing data is high enough to potentially affect results, use methods beyond simply discarding incomplete ecords-eg,inverse-probability-of-missingness weighting or multiple imputation.
Assess and handle sparse-data bias in ratio estimates with methods developed for that purpose.
If the outcome frequency is high, report risk ratios or risk differences instead of odds ratios.
Assess additive interactions even if your model is multiplicative.
本文来自:
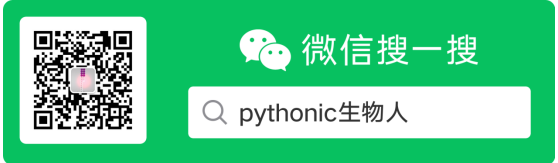